Machine Learning(ML) or automatic learning is a branch of artificial intelligence that allows computers to learn autonomously and perform certain actions or tasks without the need for specific programming. This is achieved through the development of algorithms that enable pattern learning and, consequently, decision-making.
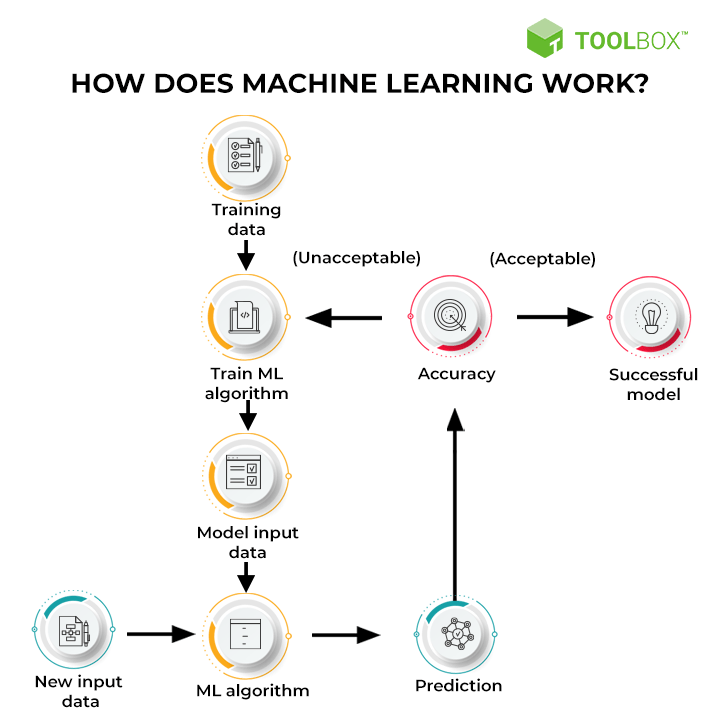
The concept of machine learning emerged in the 1950s. In the article “Computing Machinery and Intelligence” published by Alan Turing, the discussion about the ability of machines to learn is introduced, although it did not become popular until the 1990s.
The technology of digital twins utilizes algorithms of machine learning to process large amounts of data and identify data patterns. Thanks to tools based on the finite element method (FEM) and computational fluid dynamics (CFD), it is possible to accurately replicate various processes in industrial plant equipment and components. This allows for the evaluation of the process itself from an operational standpoint and the assessment of the thermal-structural behavior of the equipment.
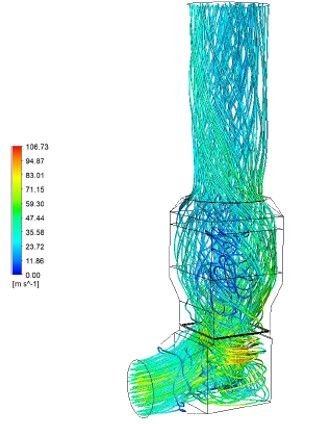
If we focus on the specific case of a digital twin, the first step in the supervised machine learning process involves the collection of real operational data from the plant containing relevant phenomena from an operational standpoint of the component (normal operation events, stops, startups, upsets, etc.) to ensure that the virtual twin offers a faithful virtual representation and, therefore, predictions about the real model are sufficiently accurate.
In this process, it is essential to have tools such as the use of Big Data to provide power, data storage capacity, and analysis capabilities to handle the large volume of data that can be generated, considering the immense number of signals that may need to be processed in each TAG of equipment and that may sometimes require minute precision of signals from the plant.
Once the starting data is selected, it is necessary to preprocess them by eliminating possible noise, as well as anomalous signals.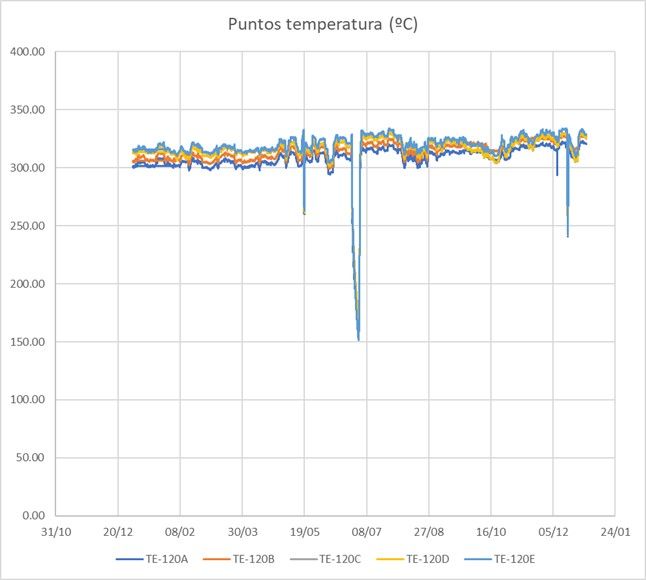
This selection of collected data is used in numerical simulations where the twin calculation model will be continuously fed. In this process, the proper adjustment of the calculation model is crucial to minimize the difference between predictions and actual responses of the equipment.
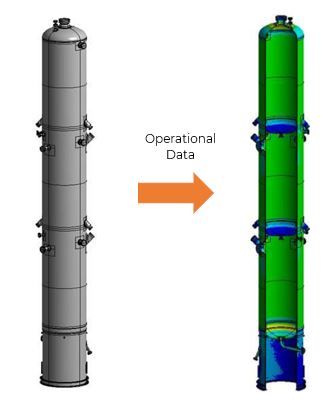
On the other hand, it is important to validate and adjust the calculation model using a series of additional real data not included in the initial learning dataset until the model is fully validated. This validation and adjustment cycle is an iterative and continuous process, as the model may need updating with new data to adapt to operational changes that may be implemented in the equipment.
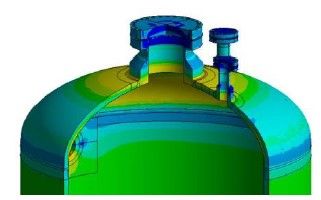
Thanks to the availability of supercomputing resources today, it has been possible to reduce the calculation times of simulations necessary for model learning, allowing for real integration and development.
The combination of the digital twin and machine learning provides a powerful tool. The ability to continuously monitor critical assets through the implementation of a digital twin enables the deployment of predictive maintenance based on anticipation and detection of possible equipment failures, quantification of remaining life, as well as damage from operation cycles, identification of inefficient behavior patterns, and thus, operational optimization of the equipment in question.
At CADE, we are fully aware that the implementation of the digital twin in plants and refineries is one of the keys to the transformation of current industrial processes and is one of the steps that will need to be taken in the energy transition process as a point of improving energy efficiency.
In the context of the digital twin, knowing how to take advantage of available operational data is synonymous with cost reduction and optimization. Our presence in industrial plants over the years, along with extensive experience in industrial processes, has allowed us to acquire key know-how in handling and interpreting the operational data needed to feed machine learning algorithms.
All of this, combined with our recognized expertise in the field of advanced simulation and our own supercomputing resources, positions us as leaders in developing and implementing digital twins.
At CADE, we not only have the ability to generate precise and efficient calculation models needed to reproduce virtual replicas and implement machine learning, but also, thanks to our extensive experience in finite element analysis and fluid dynamics, we have the capacity to calibrate and adjust these models, ensuring the development of reliable digital twins.
Further information:
For any question or further information request aboud our services and technologies, please complete the following form: